

So we set off to look for solutions - starting with considering what key datasets we could build that would be used by the whole team to save time and improve consistency. It struck me as I reviewed work that the SQL gymnastics my team members were each performing were at once both impressively masterful, but also disappointingly inefficient. Applying business logic, choosing levels of aggregation, grouping and transforming fields, checking data quality and cleaning data - these are all crucially important tasks, but they are also enormously time-consuming not to mention prone to error and inconsistency if they are done anew for each piece of analysis. They could do it, but they were doing it independently. In short, we need to make our data ‘analytics friendly’ (thank you to Rishi Nalin Kumar from whom I picked up this term).Ī few years ago, I recognized that this stage of data preparation, to make it ‘analytics friendly’, was becoming a challenge for my team of data scientists. And as we expand and transform our business, we are always looking at how we cut and slice that data to make sure we’re keeping up with the questions we are trying to answer. As we launch new features and develop new products, we instrument our product to capture the right data, transform it into something usable for analysis or experimentation, and build pipelines to keep it coming. At Spotify, we have a continuously evolving and growing volume of data at our fingertips. The data scientists on the team spend a good chunk of time on that old favorite that never seems to go away - data preparation. Our work includes exploratory analysis, experimentation, metric setting, building dashboards, user testing, ethnography, and surveys.
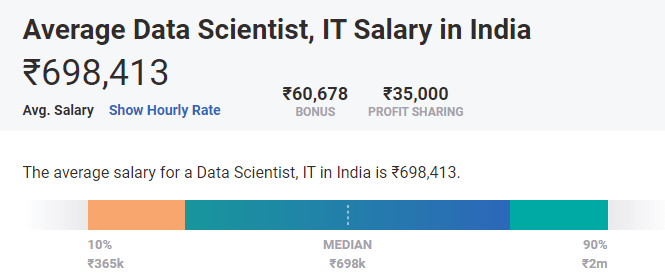
We’re a multi-disciplinary team that covers both qualitative user research and data science, as per the Spotify model. Our role is to provide guidance that enables the product and business teams we work with to meet their objectives through evidence-based decision-making and customer focus. Since 2016 I’ve led the Insights team for Spotify’s advertising business. Peter Gilks, Senior Director of Insights, Spotify Advertising
